Imagine spending months aligning on your product strategy. You’ve stocked up, trained your team, built your marketing calendar, and locked in supplier orders. Then the forecast turns out wrong. Not slightly, but completely off.
Demand drops where you expected a spike. A promotion performs, but you’re out of stock halfway through. Inventory sits where no one’s buying, and customers wait where nothing’s available.
This isn’t just another mistake. It eats margin, throws teams into fire-fighting mode, and leaves leadership questioning the plan.
We break down what demand forecasting accuracy actually means, what makes it hard to get right, and what steps you can take to fix it.
What Is Demand Forecasting Accuracy?
Demand forecasting accuracy measures how close your forecasted demand is to what customers actually bought during a specific period.
It’s a key metric for evaluating if your demand planning is working or simply setting you up for inventory headaches.
High accuracy means your sales forecast closely matches real demand. This keeps your inventory levels balanced, avoids tying up cash in unsold stock, and prevents you from running out when demand spikes.
Low accuracy means you're either overordering or underordering, both of which hurt margins and customer experience.
Shopify stores usually track accuracy weekly, monthly, or quarterly and look at it across SKUs, categories, or channels. It’s especially important when planning for promotions, seasonal peaks, or new product launches.
Now, there are two parts to it
- Forecast accuracy measures how close your predicted demand is to actual demand
- Forecast precision, on the other hand, refers to how consistently your forecasting method performs over time
You can have a forecast that’s accurate one month and way off the next. That’s where precision comes in. It helps ensure your forecasting process is reliable, not just a coincidence.
Demand Forecasting Accuracy Metrics and Scenarios
Below are the most common metrics used to measure demand forecast accuracy, each with its own strengths depending on what you're tracking. These metrics focus on operational accuracy.
1. Mean absolute percentage error (MAPE)
MAPE shows the average size of your forecast errors as a percentage of actual demand.
- Formula: Average of (|Forecast − Actual| ÷ Actual) × 100
- Pros: Easy to understand, works across SKUs and categories
- Cons: Can produce inflated values when actual demand is very low (denominator sensitivity)
- Benchmarks:
- <10% = high accuracy
- 10–20% = moderate
- Above 20% = low accuracy
2. Mean absolute error (MAE)
MAE gives the average size of your forecast errors in absolute terms (e.g., units, dollars), not percentages.
- Use cases: Great when actual volumes matter more than error rates
- Interpretation: A lower MAE means your forecasts are, on average, closer to the actual demand. It’s simple and unaffected by outliers.
3. Mean squared error (MSE)
MSE squares each error before averaging, which means big mistakes have more visibility.
- Why it’s used: Ideal when it’s critical to avoid large forecast misses (e.g., in high-cost or limited-supply products)
- When to use it: If you care more about avoiding large misses than small fluctuations
Which one should you use?
- MAPE gives a percentage-based accuracy snapshot, ideal for comparing across categories
- MAE offers a simple, volume-focused view, especially useful for tracking forecast performance over time
- MSE is best for identifying and correcting large, costly deviations
Common Challenges in Achieving Demand Forecasting Accuracy
Before you work towards improving your forecasting accuracy, it’s important to understand the challenges that hold it back.
1. Incomplete or poor-quality data
If the input is bad, the forecast is worse. Inaccurate, missing, or inconsistent data is one of the most common reasons demand forecasts miss the mark.
Legacy systems and excel-based methods often have data stored in silos. One team has last year’s sales in a spreadsheet, another has supplier orders buried in an email thread, and promotions live in someone’s head.
This disconnect leads to gaps in reading historical demand patterns and skews your forecasts from the start.
Human errors add another layer. Manual data entry, copy-paste mistakes, and outdated SKUs make it harder to trust the numbers you're using.
When data isn’t clean or complete
- You miss demand spikes caused by promotions or stockouts
- You over-rely on default trends that no longer reflect reality
- You can’t run item-level or channel-level forecasts with confidence
Without complete and consistent data, even the best forecasting model fails.
2. Market volatility and external factors
Forecasting is not done in a vacuum. External factors constantly shift demand in ways historical data cannot predict.
Seasonality plays a major role. Holiday peaks, back-to-school periods, or even unexpected weather changes create large swings that must be factored in. If seasonality patterns are not updated regularly, forecasts quickly fall behind.
Consumer trends also change fast. Viral products, shifting preferences, and economic conditions can spike or drop demand without much warning. Forecasts built on old behavior patterns fail to capture these shifts in time.
Supply chain disruptions also affect demand. New tariffs, shipping delays, and raw material shortages all affect product availability and customer expectations. Even a perfect demand forecast won’t work if the supply side cannot react.
Common signs of volatility include
- Forecasts that miss seasonal peaks or drops
- Sudden inventory shortages despite strong sales forecasts
- Overreactions to short-term consumer behavior
Staying accurate means forecasts must be adjusted frequently to factor in real-time changes across markets, consumer behavior, and supply chains.
3. Siloed teams
Most teams aren't short on data, they're short on communication. Sales is forecasting based on upcoming campaigns. Supply chain planning is based on historical averages. Finance has its own view rooted in cost controls. And none of these teams are talking to each other.
What you end up with is three forecasts, three sets of assumptions, and one confused ops team trying to figure out what to actually stock.
This isn't a software issue. It's a collaboration issue. Demand planning doesn't work when it's run in isolation.
If marketing doesn’t share campaign calendars, or sales doesn’t flag B2B deals that could skew volume, the final forecast is missing key context. Everyone has part of the truth, but no one has the full picture.
Without cross-functional input, the forecast reflects past trends, not future trends.
4. Sales vs. supply chain misalignment
Sales forecasts can feel like a wish list. Supply chain forecasts lean more toward worst-case scenario prep. Somewhere between those two is actual demand, but companies rarely find it if these teams aren't aligned.
Sales team wants enough inventory to avoid lost revenue, especially during product launches and promotions. Supply chain wants to avoid dead stock sitting in warehouses.
So supply cuts the numbers, sales bumps them up again, and the forecast becomes a tug of war that solves nothing.
It’s not just a difference in targets, it’s a difference in mindset. Sales plans for upside. Supply chain plans for risk. If they don’t meet in the middle, the business ends up overreacting, either over-ordering “just in case” or understocking and missing revenue.
No alignment means demand signals are filtered through internal bias, not customer reality.
5. Accountability issues
Sometimes the issue isn’t the forecast itself. It’s the fact that no one wants to own it.
People inflate numbers to secure more stock. Others lowball to keep expectations manageable. Some don’t update their inputs at all because they assume “someone else is handling it.” And when a forecast misses badly, the finger-pointing starts.
This isn’t just a leadership issue. It's a culture issue. If teams get penalized for forecasting errors but not rewarded for transparency, the safest move is to play defense, not accuracy.
Forecasting becomes a political tool. The numbers reflect agendas, not demand. And over time, trust in the forecast erodes. People stop relying on it, and planning turns reactive instead of proactive.
Fixing this isn’t about new tools. It’s about creating a process where people make decisions based on data, not by what looks good on paper.
How to Improve Demand Forecasting Accuracy (Best Practices)
Improving forecasting accuracy is a continuous process. While you’re in this process, here are a few best practices you should follow.
1. Start with high-quality, clean data
Bad data isn’t just a minor issue. According to Gartner, poor data quality costs companies an average of $12.9 million every year.
When your base data is messy; duplicate SKUs, inconsistent naming, missing sales periods, your forecast becomes a shot in /the dark. You're feeding the model noise and hoping it spits out a signal.
Clean, reliable data gives you actual visibility into demand patterns. It shows what sold, when, where, and how fast. To get there, you need
- Real-time inventory data
- SKU consistency across all systems
- Sales history that includes promo periods and stockouts
- Clean separation between low demand and low availability
The easiest way to get this right is to sync your systems. POS, inventory, and order data should flow into one place.
Prediko connects all your sales channels and warehouses, so your data updates in real time. You get one dashboard that shows what’s selling, where you’re overstocked, and when to reorder.
2. Choose the right forecasting model
Not all forecasting models work the same way, and they shouldn't. What works for a predictable SKU with three years of clean data won’t help with fast-moving products that spike on TikTok.
The model you choose needs to match the shape of your demand. If you're using the wrong one, even the cleanest data won’t save you.
Here’s how the most-used models actually work, and when to use them.
Time-series forecasting (eg, ARIMA, exponential smoothing)
This model is all about looking backward to move forward. It studies your past demand patterns, identifies the seasonality, trends, or cycles in the data, and projects those patterns into the future.
Time series works best when your product demand is relatively stable or has a clear rhythm. If you’ve got two or more years of consistent sales history and not too much chaos in between, this model handles the job.
What makes it strong
- It catches repeating behavior without overcomplicating
- It's transparent, you can usually see why it made a certain prediction
- Works great for short- to medium-term planning where the past really does repeat itself
It’s not made for surprises. Throw in a one-off promo, stockout, or influencer spike, and the forecast may be way off. This model doesn't ask why demand changed. It just assumes what happened before will happen again.
Regression models
This one is built for cause-and-effect. You’re not just tracking what sold, you’re tracking what pushed it. A regression model connects demand to outside variables, price, weather, ad spend, and even events like school holidays or market shifts.
Let’s say demand for umbrellas goes up when it rains. A regression model would spot that link. The next time there's rain in the forecast, it knows to expect a bump in sales, even if your last few weeks were dry.
Best used when:
- External variables clearly move your demand
- You want to simulate different scenarios (like price increases or budget cuts)
- You're managing complex categories with lots of dependencies
You’ll need more than just sales data for this. The model is only as good as the quality and range of your input variables. If the pricing data or weather feed is off, so is your forecast.
Machine learning-based forecasting
This is the model you reach for when nothing else can handle your complicated data. ML doesn’t need a clean, single-season trend. It can handle dozens of variables, missing data, channel splits, and even weird spikes caused by shipping delays.
But, how does AI improve demand forecasting accuracy? It adapts. These models learn as they go. If something shifts, like a new product line picking up traction faster than expected, it adjusts quickly, often without needing you to retrain the whole system manually.
Use machine learning if
- You have a lot of SKUs with inconsistent behavior
- Forecasting happens at scale, hundreds or thousands of items across regions
- You’re juggling data from multiple sources: sales, marketing, supply, and external inputs
However, you still need to train it, monitor it, and feed it good data regularly. But if your forecasting problems are too complex for rules and averages, this model doesn’t just survive, it performs.
Prediko automatically pulls accurate data from your Shopify store, keeps training itself on your sales patterns, and runs the analysis without any manual intervention.
Wish to dive deeper? Explore four essential forecasting types used by D2C eCommerce brands
3. Encourage cross-functional collaboration
Forecasting falls apart when it’s treated like one team’s job. Sales, marketing, finance, and supply chain all see different sides of demand, and when those sides don’t connect, the forecast ends up incomplete.
Demand planning should be a shared responsibility, not a handoff between departments. Each team brings something critical to the table
- Sales brings direct customer insight from the ground
- Marketing knows which campaigns will drive demand and when
- Finance sets constraints around cost, margin, and cash flow
- Supply chain understands lead times and fulfillment timelines
When these perspectives come in early, the forecast is grounded in reality,
One way companies put this into practice is through strong S&OP (Sales and Operations Planning) alignment. And S&OP isn’t just a recurring meeting to swap updates. It’s where teams commit to one shared plan, challenge assumptions, and make sure decisions are backed by data.
Companies that take S&OP seriously see fewer last-minute orders, fewer missed targets, and a lot less blame when things don’t go as planned.
4. Monitor, evaluate, and adjust forecasts regularly
Forecasts aren’t permanent. They’re only as good as the last time you updated them. If demand shifts and you’re still working off last month’s plan, the numbers start to slip fast.
That’s why rolling forecasts matter. You update them as new data comes in. It could be a spike in sales, a supplier delay, or a promo that outperformed. Whatever it is, you’re not waiting for next quarter’s meeting to make a change, you’re fixing it as it happens.
This only works if you’re comparing what you expected to what actually happened. Look at the difference between forecasted and actual sales. Where were you off? Which SKUs are always misfiring? Are you over-forecasting certain channels and underestimating others? These gaps are signals. They tell you where to dig in.
The best teams build that review into their routine. Weekly or monthly, they check how the forecast held up, talk through what changed, and adjust the next one accordingly.
Prediko helps here by showing actual vs forecasted sales in one place, so you don’t have to dig through spreadsheets to spot gaps. It learns your sales spikes and dips over time and flags when patterns shift, which means your next forecast already starts closer to the mark.
Using Technology & Advanced Tools for Demand Forecasting Accuracy
Technology helps increase forecasting accuracy by multiple folds.
Healf, a UK retailer, achieved a 75% ROI by using Prediko for accurate demand forecasting. With the right systems in place, they avoided stockouts, saved 10 hours per week, and improved the shopping experience.
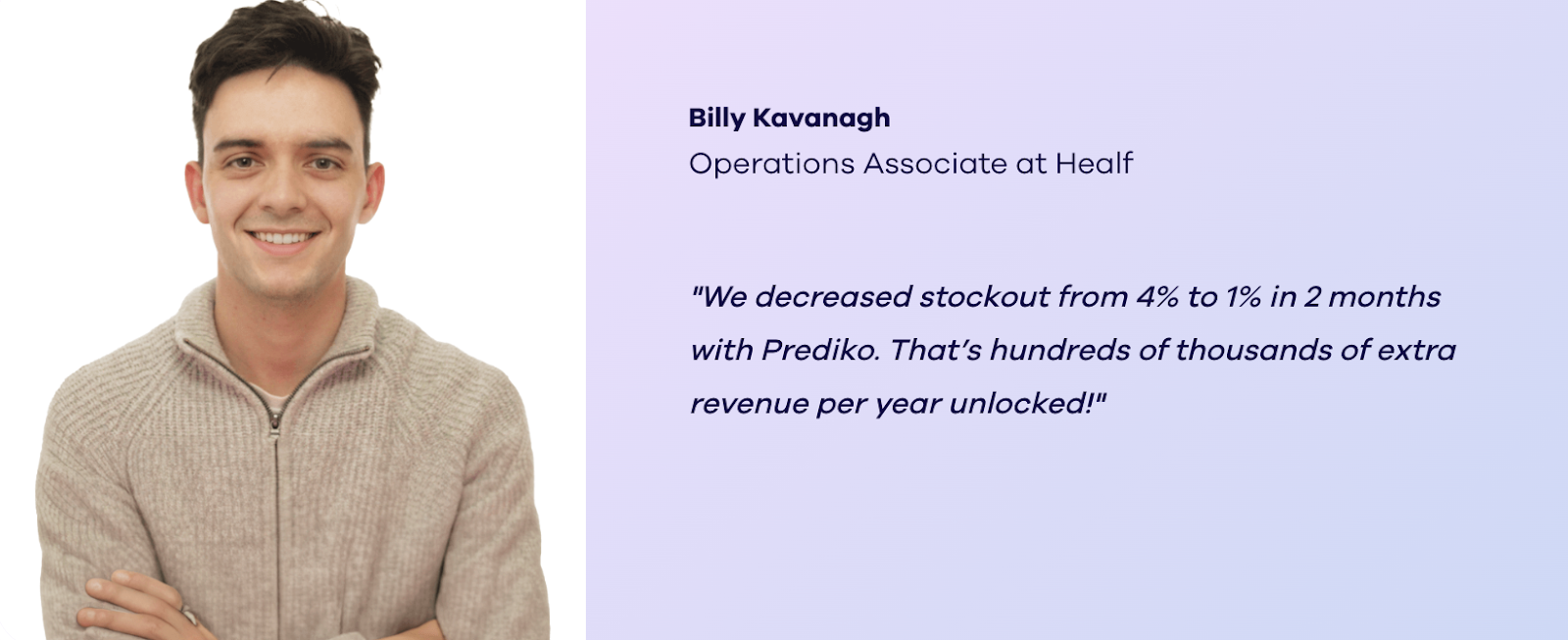
You can do the same and go even further with the right tools and technology. Here are a few that make it possible.
1. AI and predictive analytics
At the core, AI-powered forecasting software uses machine learning to analyze past sales, find patterns, and adjust predictions based on what’s happening right now. They don’t just look at what sold last year during Black Friday, they also factor in safety stock to accommodate spikes, supplier lead times, and product launches that might shape what happens this time around.
Here’s what goes into most predictive forecasting setups
- A learning engine that trains on historical data and adjusts as it sees new results
- External data inputs, like holidays, pricing shifts, promotions, weather, or web traffic
- An anomaly detection layer that flags outliers and demand spikes before they skew the data
- A feedback loop that compares forecasted vs actuals and self-corrects over time
This software can work across thousands of SKUs without breaking. They don’t get overwhelmed by noisy data, and they don’t forget to adjust for last week’s stockout or delayed shipment.
In the end, the benefit is simple: better forecasts, less firefighting. You don’t need to manually recalculate after every change. The system keeps learning, so your numbers keep improving.
That’s exactly how Prediko works. It learns from your historical data, allows adjustments for real-world factors like holidays and promotions, and self-corrects with every forecast. It even flags anomalies and demand spikes before they throw your numbers off.
Impressed by what AI can do? Learn how AI forecasting can be implemented for your Shopify brand.
2. Demand sensing vs. traditional forecasting
Traditional forecasting looks at the past to predict the future. It uses historical sales, seasonal patterns, and planned events to estimate demand weeks or months ahead. It’s useful for long-term planning but struggles when things change fast, like when a product suddenly goes viral or a shipment gets delayed.
Demand sensing works differently. It focuses on what’s happening right now.
It uses daily data from POS systems, inventory feeds, online traffic, social signals, and supply chain updates to adjust forecasts in near real-time. The goal is not to replace long-term forecasts, but to correct them with short-term signals that show what’s actually unfolding.
For businesses that deal with volatile demand, fast-moving products, or high inventory risk, demand sensing helps you course-correct before problems stack up. It’s not about predicting a quarter. It’s about fixing the next seven days.
3. Integrating forecasting with ERP, CRM, and POS systems
Forecasting only works when it’s pulling data from the right places.
If your sales data is in one platform, inventory in another, and customer info stuck in a CRM no one checks, your forecast is stitched together with gaps. Integrating forecasting with ERP, CRM, and POS systems brings all those signals into one place.
This kind of setup gives you a single source of truth. Your forecast is built on what customers are actually doing and what inventory is really available, not just what happened last year.
- ERP gives you supplier lead times, current stock levels, and open orders
- CRM adds sales data, customer intent, and order details
- POS systems track real-time transactions and returns across stores or regions
When all of this feeds into your forecasting engine, you’re better prepared for predicting demand accurately.
Prediko brings it all together by pulling in orders, sales, purchase orders, POS data, WMS, and even 3PL updates into one place. So you’re not patching reports across apps, you’re working from one live dashboard that’s always up to date.
How to Measure Demand Forecasting Accuracy Continuously
There are two things that make accurate forecasting sustainable: setting the right performance metrics and using those to improve how you plan. Most brands track accuracy once the quarter wraps, but that view is limited.
By then, stockouts have already happened, overorders have already tied up cash, and customer demand has already shifted.
Accuracy needs to be monitored in real time, tracked at the right level (SKU, channel, region), and used to adjust the next forecast.
1. Key KPIs to track
The following KPIs give you a strategic view of how well accurate forecasting supports the bigger business goals.
Forecast bias
This measures the direction of your forecasting error. It shows whether you consistently forecast too high or too low.
Over time, a biased forecast can distort purchasing and inventory plans.
Formula: Forecast Bias during specific period (%) = (Forecasted demand - Actual demand) ÷ Actual demand × 100
- A positive bias means you’re over-forecasting (ordering more than needed)
- A negative bias means you’re under-forecasting (not stocking enough)
- A result close to zero indicates low bias, your forecasts are generally balanced
Bias is especially useful when you break it down by product type, region, or time period. A brand may find that it over-forecasts new product launches but under-forecasts during seasonal peaks. Without bias tracking, these patterns stay hidden.
Inventory turnover ratio
While not a forecasting metric on its own, the turnover ratio shows whether your forecasts are helping you move stock at the right pace. If you're forecasting well but turnover is slow, it points to issues in sell-through or overordering.
Formula: Inventory Turnover = Cost of Goods Sold ÷ Average Inventory
- A high ratio suggests healthy inventory flow, assuming stockouts aren’t common
- A low ratio could point to consistent over-forecasting or slow-moving inventory
- When matched with forecast accuracy data, it helps explain why you’re overstocked or understocked
Used together, bias and turnover tell a fuller story. You’re not just measuring how far off you were, but whether that miss led to inventory decisions that hurt performance.
2. Build a continuous improvement loop
Tracking metrics is useful, but only if you act on what they reveal. A continuous improvement loop takes accurate data and turns it into input for the next forecast cycle.
This isn’t about reviewing numbers after the fact. It’s about using mistakes to improve future decisions.
Start with a short-cycle review, weekly or biweekly. Look at the variance between forecasted and actual demand, then investigate what caused the gap.
Maybe a promo didn’t perform, a supplier delay affected availability, or a new SKU sold faster than expected.
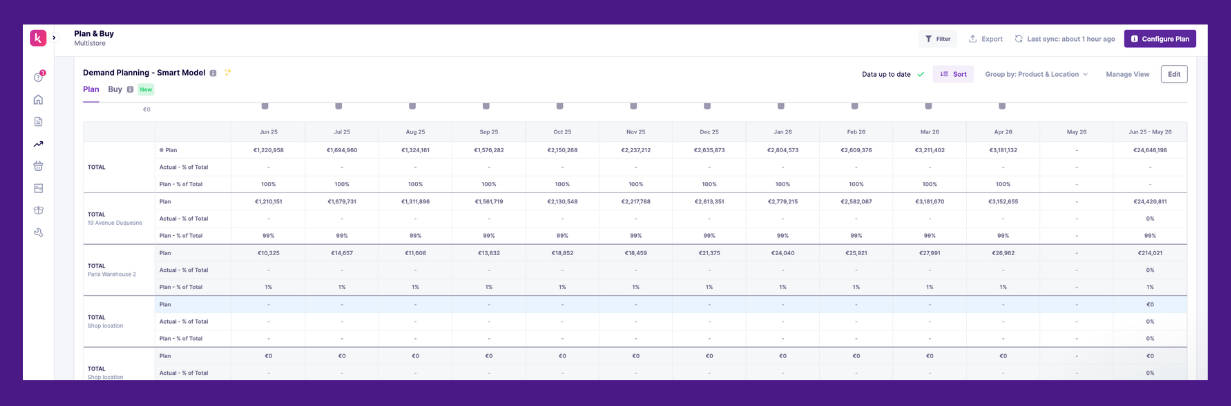
Prediko makes this easy by showing actual vs forecasted numbers directly in your dashboard, broken down by product, location, and SKUs. It’s built to support fast, insight-driven reviews, whether you run them weekly, biweekly, or whenever your team’s ready to act.
Remember, dashboards help only if they support action. Too often, they become passive reporting tools. What matters is that teams use them to discuss trade-offs, refine assumptions, and test ideas.
Prediko allows you to make purchasing decisions while reviewing performance so far, allowing you to stock based on real data.
How Prediko Helps Improve Demand Forecasting Accuracy
Most demand forecasting apps stop at projections. They give you a number and leave the rest up to you. But the real challenge isn’t just predicting demand, it’s adjusting when things change, spotting risks early, and making confident decisions without rebuilding your entire plan.
That’s where Prediko fits in. It connects your forecast to your actuals, your sales data to your inventory movements, and your long-term targets to the actions you're taking week to week.
Forecasting stops being a back-office function and becomes part of how you run the business day to day.
Here's how Prediko makes that possible.
- AI demand planning: Its AI analyzes your sales, seasonality patterns, and growth trends to build an accurate forecast across products, locations, and sales channels aligned with your revenue targets. It updates every time new sales or stock flows in and displays forecasts alongside current sell-through, so you can validate them in context.

- Real-time sync with Shopify: Pulls all sales and inventory data from Shopify in real time, so that forecasts use current, accurate inputs, eliminating errors from manual typing or copy-pasting.
- AI supply planning: Calculates exact reorder quantities and timing using lead times, safety stock, and demand forecasts. “Buy Now” and “Buy Before” alerts to prevent stock imbalances.

- Purchase order management: Creates POs based on real-time sales data and AI forecasts so that reorders match actual demand. Automates order timing, reducing overstock or stockouts from misjudged needs.
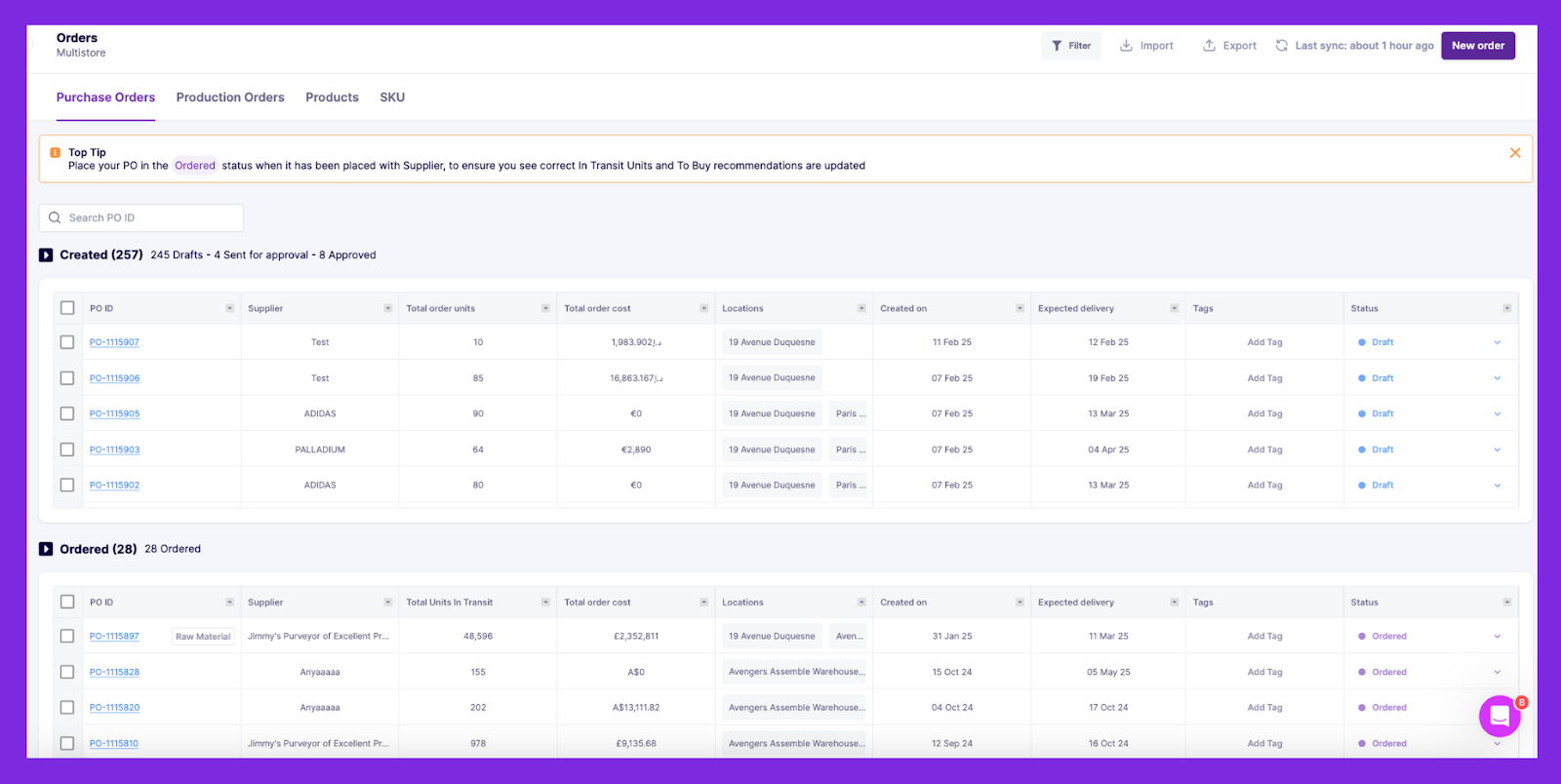
- Raw materials management: It ties raw material needs to finished goods forecasts, ensuring production aligns with demand predictions. Imports eliminate manual errors, simplify material planning.

- 60+ integrations: It connects with WMS, 3PLs, and platforms to pull real-time data. The unified inputs from multiple sources reduce errors from fragmented data.
- Analytics & reports: Monitors KPIs like stockouts, excess inventory, and upcoming POs for data-backed adjustments to forecasts. Daily and weekly reports to flag discrepancies so that predictions stay in line with current trends.

Start your 14-day free trial of Prediko and see how accurate your forecasting can get.
FAQs
1. How do you determine accuracy in forecasting?
By comparing forecasted values to actual sales using metrics like MAPE, MAE, or Forecast Bias.
2. How do you measure demand planning forecast accuracy?
Use historical data to calculate error rates between forecasted and actual demand at the SKU or category level.
3. What is a good demand forecast accuracy?
There’s no universal benchmark. Most companies aim between 70% and 90%, depending on product type, lead time, and data quality.
4. Why is forecast accuracy important in demand planning?
Demand forecast accuracy is important because it directly affects inventory levels, cash flow, and service rates. Poor accuracy leads to overstock, stockouts, or both.